Coupled DAE Problems
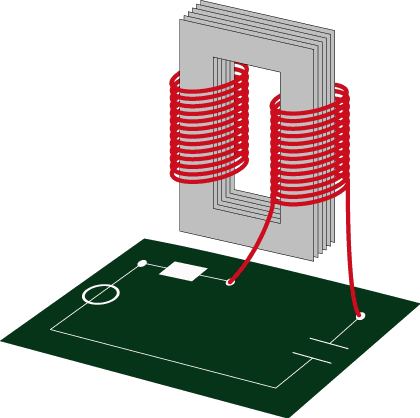
A circuit (DAE model) coupled to a magnetostatic field device (PDE model)
Coupled Problems of differential-algebraic equations (DAEs) arise typically from either multiphysical modeling (e.g. in circuit simulation with heating) or from refined modeling, where crucial parts of the original problem are replaced by a better, but computational more expensive model (e.g. circuits refined by field models). Furthermore splitting methods may turn a monolithic DAE problem into coupled subproblems, e.g. because of different time scales (multirate). In any case the DAEs arise from network approaches or space-discretization of PDAEs (Partial Differential Algebraic Equations).
Often the coupled equations have quite different properties, i.e., symmetries, definiteness or time scales. Thus the coupled system must be analyzed (e.g. the index) and tailored methods have to be developed (e.g. dynamic iteration).
Details
Publications
- 2024
5263.
Zaspel, Peter; Günther, Michael
Data-driven identification of port-Hamiltonian DAE systems by Gaussian processes
Preprint
20245262.
Zaspel, Peter; Günther, Michael
Data-driven identification of port-Hamiltonian DAE systems by Gaussian processes.
20245261.
Kapllani, Lorenc; Teng, Long
Deep learning algorithms for solving high-dimensional nonlinear backward stochastic differential equations
Discrete and continuous dynamical systems - B, 29 (4) :1695–1729
2024
Herausgeber: AIMS Press5260.
Ackermann, Julia; Jentzen, Arnulf; Kuckuck, Benno; Padgett, Joshua Lee
Deep neural networks with ReLU, leaky ReLU, and softplus activation provably overcome the curse of dimensionality for space-time solutions of semilinear partial differential equations
arXiv:2406.10876 :64 pages
20245259.
Kossaczká, Tatiana; Jagtap, Ameya D; Ehrhardt, Matthias
Deep smoothness weighted essentially non-oscillatory method for two-dimensional hyperbolic conservation laws: A deep learning approach for learning smoothness indicators
Physics of Fluids, 36 (3)
2024
Herausgeber: AIP Publishing5258.
Kossaczká, Tatiana; Jagtap, Ameya D; Ehrhardt, Matthias
Deep smoothness WENO method for two-dimensional hyperbolic conservation laws: A deep learning approach for learning smoothness indicators
Physics of Fluid, 36 (3) :036603
2024
Herausgeber: AIP Publishing5257.
Kossaczká, Tatiana; Jagtap, Ameya D; Ehrhardt, Matthias
Deep smoothness WENO method for two-dimensional hyperbolic conservation laws: A deep learning approach for learning smoothness indicators
Physics of Fluid, 36 (3) :036603
2024
Herausgeber: AIP Publishing5256.
Stiglmayr, Michael; Uhlemeyer, Svenja; Uhlemeyer, Björn; Zdrallek, Markus
Determining Cost-Efficient Controls of Electrical Energy Storages Using Dynamic Programming
Journal of Mathematics in Industry
20245255.
Ehrhardt, M.; Kruse, T.; Tordeux, A.
Dynamics of a Stochastic port-{H}amiltonian Self-Driven Agent Model in One Dimension
ESAIM: Math. Model. Numer. Anal.
20245254.
Efficient and Simple Extraction Protocol for Triterpenic Acids from Apples
Journal of Chemical Education, 101 :2087-2093
April 2024
Herausgeber: ACS5253.
Santos, Daniela Scherer; Klamroth, Kathrin; Martins, Pedro; Paquete, Luís
Ensuring connectedness for the Maximum Quasi-clique and Densest $k$-subgraph problems
20245252.
Holzenkamp, Matthias; Lyu, Dongyu; Kleinekathöfer, Ulrich; Zaspel, Peter
Evaluation of uncertainty estimations for Gaussian process regression based machine learning interatomic potentials.
20245251.
Gaul, Daniela
Exact and Heuristic Methods for Dial-a-Ride Problems
Dissertation
Dissertation
Bergische Universität Wuppertal
20245250.
Lyu, Dongyu; Holzenkamp, Matthias; Vinod, Vivin; Holtkamp, Yannick M.; Maity, Sayan; Salazar, Carlos R.; Kleinekathöfer, Ulrich; Zaspel, Peter
Excitation Energy Transfer between Porphyrin Dyes on a Clay Surface: A study employing Multifidelity Machine Learning.
20245249.
Kienitz, Jörg
Exciting times are ahead - Gaussian views and yield curve extrapolation
Wilmott, 2024 (134) :46–50
2024
Herausgeber: Wilmott Magazine5248.
[german] Zeller, Diana; Bohrmann-Linde, Claudia
Falschinformationen in Videos? Mit dem Konzept KriViNat die Kompetenz der Informationsbewertung stärken
In Bohrmann-Linde, C.; Gökkus, Y.; Meuter, N.; Zeller, D., Editor, Band Netzwerk Digitalisierter Chemieunterricht. Sammelband NeDiChe-Treff 2022
Seite 9-15
Herausgeber: Chemiedidaktik. Bergische Universität Wuppertal
2024
9-155247.
Bartel, Andreas; Schaller, Manuel
Goal-oriented time adaptivity for port-{H}amiltonian systems
20245246.
Schäfers, Kevin; Finkenrath, Jacob; Günther, Michael; Knechtli, Francesco
Hessian-free force-gradient integrators
20245245.
Hosfeld, René; Jacob, Birgit; Schwenninger, Felix; Tucsnak, Marius
Input-to-state stability for bilinear feedback systems
SIAM Journal on Control and Optimization, 62 (3) :1369-1389
20245244.
Jamil, Hamza
Intrusive and non-intrusive uncertainty quantification methodologies for pyrolysis modeling
Fire Safety Journal, 143 :104060
2024
ISSN: 0379-71125243.
Botchev, M. A.; Knizhnerman, L. A.; Schweitzer, M.
Krylov subspace residual and restarting for certain second order differential equations
SIAM J. Sci. Comput., 46 (2) :S223-S253
20245242.
Hastir, Anthony; Jacob, Birgit; Zwart, Hans
Linear-Quadratic optimal control for boundary controlled networks of waves
20245241.
Xu, Zhuo; Tucsnak, Marius
LQR control for a system describing the interaction between a floating solid and the surrounding fluid
Mathematical Control and Related Fields, 14(4) :1477-1500
Dezember 20245240.
Costa, G Morais Rodrigues; Ehrhardt, Matthias
Mathematical analysis and a nonstandard scheme for a model of the immune response against COVID-19
Band 793
Seite 251–270
Herausgeber: AMS Contemporary Mathematics
2024
251–2705239.
Costa, G Morais Rodrigues; Ehrhardt, Matthias
Mathematical analysis and a nonstandard scheme for a model of the immune response against COVID-19
Band 793
Seite 251–270
Herausgeber: AMS Contemporary Mathematics
2024
251–270