Thermal Coupling
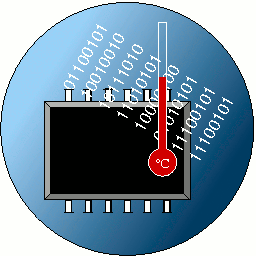
The performance of high-tech circuitry such as processors and power devices also largely depends on the thermal level. Semiconductor devices loss their ability of fast switching if the temperature increases to much. Furthermore after a critical temperature is reached the device will be destroyed. Therefore monitoring temperature and regulating cooling are important issues.
In our research, we set up simulation models for semiconductor equations and integrated circuits, which incorporate transient temperature changes in the device and heat conduction between devices. That is an electric network as well as semiconductor equations have to be equipped with an appropriate model for power transfer and heat conduction.
Since this multiphysical problem of coupled electric networks and heat conduction exhibits widely separated time scales, not only the model but also the numerical algorithms need be design to enable fast simulations. Multirate cosimulation is an good choice if the coupling is appropriately set up. Please see also: (Coupled DAEs).
Publications
- 2023
5142.
Carrillo, Jose Antonio; Totzeck, Claudia; Vaes, Urbain
Consensus-based Optimization and Ensemble Kalman Inversion for Global Optimization Problems with Constraints
, Modeling and Simulation for Collective Dynamics,Lecture Notes Series, Institute for Mathematical Sciences, NUS Band 40
20235141.
Halim, A. Abdul; others
Constraining the sources of ultra-high-energy cosmic rays across and above the ankle with the spectrum and composition data measured at the Pierre Auger Observatory
JCAP, 05 :024
20235140.
Yue, Baobiao; others
Constraints on BSM particles from the absence of upward-going air showers in the Pierre Auger Observatory
PoS, ICRC2023 :1095
20235139.
Abdul Halim, Adila; others
Constraints on UHECR characteristics from cosmogenic neutrino limits with the measurements of the Pierre Auger Observatory
PoS, ICRC2023 :1520
20235138.
Abdul Halim, Adila; others
Constraints on upward-going air showers using the Pierre Auger Observatory data
PoS, ICRC2023 :1099
20235137.
Acu, Ana-Maria; Heilmann, Margareta; Raşa, Ioan; Seserman, Andra
Convergence of linking Durrmeyer type modifications of generalized Baskakov operators
Bulletin of the Malaysian Math. Sciences Society, 46 (3)
20235136.
Jacob, Birgit; Mironchenko, Andrii; Partington, Jonathan R.; Wirth, Fabian
Corrigendum: Noncoercive Lyapunov functions for input-to-state stability of infinite-dimensional systems
SIAM J. Control Optim., 61 (2) :723-724
20235135.
Aerdker, S.; others
CRPropa 3.2: a public framework for high-energy astroparticle simulations
PoS, ICRC2023 :1471
20235134.
Günther, Michael; Jacob, Birgit; Totzeck, Claudia
Data-driven adjoint-based calibration of port-Hamiltonian systems in time domain
arXiv preprint arXiv:2301.03924
20235133.
Kossaczká, Tatiana; Ehrhardt, Matthias; Günther, Michael
Deep FDM: Enhanced finite difference methods by deep learning
Franklin Open, 4 :100039
2023
Herausgeber: Elsevier5132.
Kossaczká, Tatiana; Ehrhardt, Matthias; Günther, Michael
Deep FDM: Enhanced finite difference methods by deep learning
Franklin Open, 4 :100039
2023
Herausgeber: Elsevier5131.
Kossaczká, Tatiana; Ehrhardt, Matthias; Günther, Michael
Deep FDM: Enhanced finite difference methods by deep learning
Franklin Open, 4 :100039
2023
Herausgeber: Elsevier5130.
Kossaczká, Tatiana; Ehrhardt, Matthias; Günther, Michael
Deep finite difference method for solving Asian option pricing problems
Preprint IMACM
2023
Herausgeber: Bergische Universität Wuppertal5129.
Kossaczká, Tatiana; Ehrhardt, Matthias; Günther, Michael
Deep finite difference method for solving Asian option pricing problems
Preprint IMACM
2023
Herausgeber: Bergische Universität Wuppertal5128.
Kapllani, Lorenc; Teng, Long
Deep Learning algorithms for solving high-dimensional nonlinear Backward Stochastic Differential Equations
Discrete Contin. Dyn. Syst. - B
2023
ISSN: 1531-34925127.
Kapllani, Lorenc; Teng, Long
Deep Learning algorithms for solving high-dimensional nonlinear backward stochastic differential equations
Discrete Contin. Dyn. Syst. - B
20235126.
Ackermann, Julia; Jentzen, Arnulf; Kruse, Thomas; Kuckuck, Benno; Padgett, Joshua Lee
Deep neural networks with ReLU, leaky ReLU, and softplus activation provably overcome the curse of dimensionality for Kolmogorov partial differential equations with Lipschitz nonlinearities in the $L^p$-sense
20235125.
Ackermann, Julia; Jentzen, Arnulf; Kruse, Thomas; Kuckuck, Benno; Padgett, Joshua Lee
Deep neural networks with ReLU, leaky ReLU, and softplus activation provably overcome the curse of dimensionality for Kolmogorov partial differential equations with Lipschitz nonlinearities in the Lp-sense
Preprint
20235124.
Ackermann, Julia; Jentzen, Arnulf; Kruse, Thomas; Kuckuck, Benno; Padgett, Joshua Lee
Deep neural networks with ReLU, leaky ReLU, and softplus activation provably overcome the curse of dimensionality for Kolmogorov partial differential equations with Lipschitz nonlinearities in the Lp-sense
Preprint
20235123.
Abdul Halim, Adila; others
Deep-Learning-Based Cosmic-Ray Mass Reconstruction Using the Water-Cherenkov and Scintillation Detectors of AugerPrime
PoS, ICRC2023 :371
20235122.
Kowol, Philipp; Bargmann, Swantje; Görrn, Patrick; Wilmers, Jana
Delamination Behavior of Highly Stretchable Soft Islands Multi-Layer Materials
Applied Mechanics, 4 (2) :514--527
2023
ISSN: 2673-31615121.
Ehrhardt, Matthias; Matyokubov, Kh Sh
Driven transparent quantum graphs
Preprint
20235120.
Ehrhardt, Matthias; Matyokubov, Kh Sh
Driven transparent quantum graphs
Preprint
20235119.
Felpel, Mike; Kienitz, Jörg; McWalter, Thomas
Effective stochastic local volatility models
Quantitative Finance, 23 (12) :1731–1750
2023
Herausgeber: Routledge5118.
Klamroth, Kathrin; Lang, Bruno; Stiglmayr, Michael
Efficient Dominance Filtering for Unions and Minkowski Sums of Non-Dominated Sets
Computers and Operations Research
2023
Herausgeber: Elsevier {BV}