Semiconductor
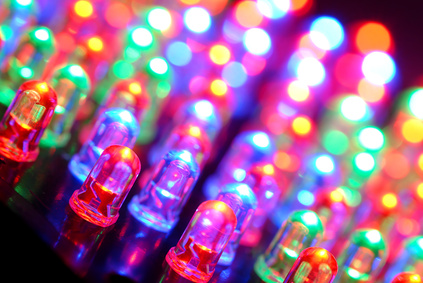
Semiconductor devices are solid state bodies, whose electrical conductivity strongly depends on the temperature and other internal properties like the so-called doping. Depending on the temperature or other internal settigns, they can be regarded as insulator or conductor. (Physically speaken: Semiconductor materials have a band gap between.. and .. electron Volt)
This property makes them extremely useful in electronics, since this property can be easily employed to use them as switches. On nowadays computerchips and prozessors, millions of semiconductor devices (especially transistors) are included in an electronic circuit. In order to use common circuit simulation tools to simualte circuits containing those devices, semiconductor devices are often reflected by compact models - subcircuits of basic elements like resistors, capacitors, inductors and current/voltage sources. Those compact models shoul rebuild the input/output behaviour of the semiconductor device.
Ongoing miniaturization and the step from miro- to nanotechnology, however, leads to more powerful prozessors and chips, since higher packing density can be achieved. On the other hand, this higher packing density and miniaturization of the devices makes parasitic effects like heating predominant. Incorporation of those effects into compact models results in large compact models to describe a single semiconductor device. This makes it desireable to include more exact distributed device models - device models based on partial differential equations - into circuit simulation.
Moreover, smaller devices are driven by smaller signals, what makes them more energy efficient. On the other hand this results in a larger noise/signal ratio, what makes inclusion of non-deterministic effects into device models interesting. All in all, this leads to the following recent question in semiconductor/circuit modelling and simulation:
- Thermal effects in semiconductor devices
- Noise in semiconductor devices (SDEs)
- Quantum Effects in semiconductor devices
- Electro-thermal coupling of optoelectronic semiconductor devices with electric circuits
- Efficient Co-Simulation of circuit/semiconductor problems (Dynamic Iteration schemes)
Former and ongoing projects
Cooperations
- Vittorio Romano, Università degli studi di Catania, Italy
- Giuseppe Ali, Universitá della Calabria, Italy
- Ansgar Jüngel, TU Vienna, Austria
- Pina Milisic, University of Zagreb, Croatia
Open subjects for theses
- Master Thesis: Two-dimensional thermal-electric simulation of semiconductor MOSFET-devices (M.Brunk)
Publications
- 2024
5292.
Vorberg, Lukas; Jacob, Birgit; Wyss, Christian
Computing the Quadratic Numerical Range
Journal of Computational and Applied Mathematics :116049
20245291.
Klamroth, Kathrin; Stiglmayr, Michael; Totzeck, Claudia
Consensus-Based Optimization for Multi-Objective Problems: A Multi-Swarm Approach
Journal of Global Optimization
20245290.
Günther, Michael; Jacob, Birgit; Totzeck, Claudia
Data-driven adjoint-based calibration of port-Hamiltonian systems in time domain
Mathematics of Control, Signals, and Systems, 36 (4) :957–977
2024
Herausgeber: Springer London5289.
Günther, Michael; Jacob, Birgit; Totzeck, Claudia
Data-driven adjoint-based calibration of port-Hamiltonian systems in time domain
Mathematics of Control, Signals, and Systems, 36 (4) :957–977
2024
Herausgeber: Springer London5288.
Günther, M.; Jacob, B.; Totzeck, C.
Data-driven adjoint-based calibration of port-Hamiltonian systems in time domain
Math. Control Signals Syst., 36 :957–977
20245287.
Günther, M.; Jacob, Birgit; Totzeck, Claudia
Data-driven adjoint-based calibration of port-Hamiltonian systems in time domain
Math. Control Signals Syst.
20245286.
Zaspel, Peter; Günther, Michael
Data-driven identification of port-Hamiltonian DAE systems by Gaussian processes
Preprint
20245285.
Zaspel, Peter; Günther, Michael
Data-driven identification of port-Hamiltonian DAE systems by Gaussian processes
Preprint
20245284.
Zaspel, Peter; Günther, Michael
Data-driven identification of port-Hamiltonian DAE systems by Gaussian processes.
20245283.
Kapllani, Lorenc; Teng, Long
Deep learning algorithms for solving high-dimensional nonlinear backward stochastic differential equations
Discrete and continuous dynamical systems - B, 29 (4) :1695–1729
2024
Herausgeber: AIMS Press5282.
Ackermann, Julia; Jentzen, Arnulf; Kuckuck, Benno; Padgett, Joshua Lee
Deep neural networks with ReLU, leaky ReLU, and softplus activation provably overcome the curse of dimensionality for space-time solutions of semilinear partial differential equations
arXiv:2406.10876 :64 pages
20245281.
Kossaczká, Tatiana; Jagtap, Ameya D; Ehrhardt, Matthias
Deep smoothness weighted essentially non-oscillatory method for two-dimensional hyperbolic conservation laws: A deep learning approach for learning smoothness indicators
Physics of Fluids, 36 (3)
2024
Herausgeber: AIP Publishing5280.
Kossaczká, Tatiana; Jagtap, Ameya D; Ehrhardt, Matthias
Deep smoothness WENO method for two-dimensional hyperbolic conservation laws: A deep learning approach for learning smoothness indicators
Physics of Fluid, 36 (3) :036603
2024
Herausgeber: AIP Publishing5279.
Kossaczká, Tatiana; Jagtap, Ameya D; Ehrhardt, Matthias
Deep smoothness WENO method for two-dimensional hyperbolic conservation laws: A deep learning approach for learning smoothness indicators
Physics of Fluid, 36 (3) :036603
2024
Herausgeber: AIP Publishing5278.
Stiglmayr, Michael; Uhlemeyer, Svenja; Uhlemeyer, Björn; Zdrallek, Markus
Determining Cost-Efficient Controls of Electrical Energy Storages Using Dynamic Programming
Journal of Mathematics in Industry
20245277.
Ehrhardt, M.; Kruse, T.; Tordeux, A.
Dynamics of a Stochastic port-{H}amiltonian Self-Driven Agent Model in One Dimension
ESAIM: Math. Model. Numer. Anal.
20245276.
Itzenhäuser, Patricia; Wachter, Ferdinand Max; Lehmann, Laura; Rajkovic, Michelle; Benter, Thorsten; Wissdorf, Walter
Dynamics of the Aspiration of Charged Droplets into a LC-ESI-MS System
Journal of the American Society for Mass Spectrometry :jasms.4c00238
September 2024
ISSN: 1044-0305, 1879-11235275.
Efficient and Simple Extraction Protocol for Triterpenic Acids from Apples
Journal of Chemical Education, 101 :2087-2093
April 2024
Herausgeber: ACS5274.
Santos, Daniela Scherer; Klamroth, Kathrin; Martins, Pedro; Paquete, Luís
Ensuring connectedness for the Maximum Quasi-clique and Densest $k$-subgraph problems
20245273.
Holzenkamp, Matthias; Lyu, Dongyu; Kleinekathöfer, Ulrich; Zaspel, Peter
Evaluation of uncertainty estimations for Gaussian process regression based machine learning interatomic potentials.
20245272.
Gaul, Daniela
Exact and Heuristic Methods for Dial-a-Ride Problems
Dissertation
Dissertation
Bergische Universität Wuppertal
20245271.
Lyu, Dongyu; Holzenkamp, Matthias; Vinod, Vivin; Holtkamp, Yannick M.; Maity, Sayan; Salazar, Carlos R.; Kleinekathöfer, Ulrich; Zaspel, Peter
Excitation Energy Transfer between Porphyrin Dyes on a Clay Surface: A study employing Multifidelity Machine Learning.
20245270.
Kienitz, Jörg
Exciting times are ahead - Gaussian views and yield curve extrapolation
Wilmott, 2024 (134) :46–50
2024
Herausgeber: Wilmott Magazine5269.
[german] Zeller, Diana; Bohrmann-Linde, Claudia
Falschinformationen in Videos? Mit dem Konzept KriViNat die Kompetenz der Informationsbewertung stärken
In Bohrmann-Linde, C.; Gökkus, Y.; Meuter, N.; Zeller, D., Editor, Band Netzwerk Digitalisierter Chemieunterricht. Sammelband NeDiChe-Treff 2022
Seite 9-15
Herausgeber: Chemiedidaktik. Bergische Universität Wuppertal
2024
9-155268.
Bartel, Andreas; Schaller, Manuel
Goal-oriented time adaptivity for port-{H}amiltonian systems
2024